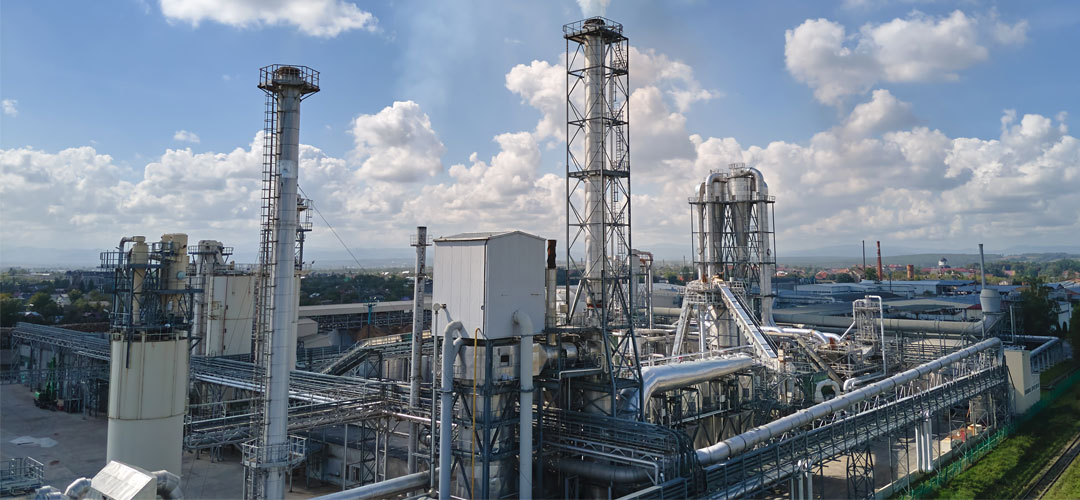
8 Real World Use Cases of Computer Vision in the Oil & Gas Industry
8 Real World Use Cases of Computer Vision in the Oil & Gas Industry
One of the key advancements of AI is in the field of computer vision, which is quickly finding its way into the oil and gas sector, where it promises to usher in a period of unprecedented innovation and economic prosperity. Artificial intelligence has already caused seismic shifts and rewritten the laws of competitiveness in numerous sectors. Businesses now seek to generate value with the help of AI systems rather than the more conventional methods that focus on humans. Organizations are racing to iteratively optimise and run AI technology at scale since AI is changing the laws of competitiveness by constructing internal capabilities, developing unique AI vision applications, and accumulating learnings through early adoption.
Several Oil and Gas companies have invested heavily in data science and machine learning (ML) and are starting to see returns on their investments. The majority of these funds have gone toward improving the company’s central production processes. In order to enhance oil well finding and precision drilling, machine learning and data analytics are currently frequently used. Oil and gas companies can reap additional benefits from the use of machine learning, artificial intelligence, and computer vision, including a lesser impact on the environment.
Let’s have a look at some of the use cases of Computer Vision in the Oil & Gas sector.
1. Extensive Pipeline Inspection for Oil or Gas
In-service or extreme hazard event assessments of large-scale infrastructure systems for condition and health status provide significant problems for operators. By utilising computer vision models, deep learning techniques may conditionally evaluate large-scale systems by drawing crucial insights from camera-based remote sensing data.
It is necessary to first do pixel-level pre-processing using conventional computer vision techniques for the images. Finally, the health of several vital parts is assessed using deep learning (DL) models (like R-CNN). Experimental results from real-world use cases have shown that DL models can pinpoint the precise position and severity of damage in a short amount of time. As a result, large-scale oil/gas pipeline assessment has an edge over traditional models in both the geographical and temporal dimensions.
2. Monitoring the Fingerboard Latches
Keeping an eye on the fingerboard latches is an important part of running a safe drilling rig and avoiding costly accidents. While most rigs already have or are installing CCTVs to provide film of the latches, this method still requires human verification of the latch locations. Real-time confirmation across several fingerboard latches is necessary to continue operations, making this an error-prone and costly challenge. Using computer vision models trained on latch open/close positions, CV solutions can give visual confirmation of latch conditions in real-time. This has significant financial benefits as it decreases the requirement for human spotters while simultaneously increasing safety.
3. Remote?Oil and Gas Production Tracking
Offshore oil and gas fields can benefit from automated and digitally documented maintenance thanks to cameras monitoring the fields in real-time. This type of technology uses machine learning to keep tabs on the status of load pumps in an effort to improve oil and gas output. Low-cost sensors and high-performance computers with distributed systems are driving the digital transformation of the oil and gas sector by allowing for the extraction of high-value information from big data at the point of collection. Large-scale video analysis is now possible without the need to attach physical sensors thanks to the multi-dimensional value and relatively inexpensive costs of cameras.
3. Checking the Danger Zone
Drilling operations are complicated, involving many individuals working in close proximity to dangerous machinery. Drill floors often have “red zones” where crews are required to keep a close eye out for potential dangers while carrying out their duties.
Machine learning models for computer vision make it possible to keep tabs on machinery and personnel in real time, where any intrusions or potentially harmful actions can be quickly identified.
4. Using Computer Vision?to Spot?Leaks
Regular infrared cameras are used in conjunction with machine vision to identify methane gas emissions. One recent use of deep learning involves the detection of methane. The automated method streamlines the analysis of leak detection while maintaining an accuracy of 95-99%. When it comes to finding methane leaks, the standard optical gas imaging (OGO) procedures are time-consuming and subjective, requiring the intervention of a human operator. Convolutional neural network (CNN) techniques for optical gas imaging in computer vision require training using methane leak photos for automatic identification.
5. Recognizing Analog Instruments Automatically
Analog gauges at power substations and other facilities can be read with the use of computer vision. Computer vision cameras are used to automatically read oil level gauges, winding temperature gauges, and SF6 gas density gauges (find the 2021 study here). Therefore, the CV algorithms use colour segmentation to identify the location of the pointers and scale marks. Such programmes help to prevent harmful mishaps and costly production halts, and they do it more faster and more precisely than humans.
6. Incorporating Computer Vision into Automated Wireline Spooling
Wirelines are used for well intervention and reservoir evaluation in the oil and gas industry. The wireline cable is usually under tension while it is spooled on a drum while the toolstring is being pulled from the well. Adequate spooling is essential to prevent cable damage, which could be disastrous. Spooling anomalies and cable position predictions (using new computer vision applications) can now be made in real time.
7. How Computer Vision helps improve Workplace Safety
Theft of expensive tools from unguarded sites, vandalism, and sabotage all pose risks to the oil and gas business and can result in leaks and other damages. Leakage that contaminates groundwater is an expensive and serious problem that can result in fines, criminal charges, and severe damage to a company’s reputation. Moreover, terrorists and saboteurs may target the oil and gas industries due to their economic significance.
Security issues, such as intrusion, may be monitored around the clock at unattended operation sites with the use of computer vision solutions?which can then immediately warn relevant authorities. Furthermore, security agents are able to focus on real dangers rather than trying to keep track of numerous screens at once thanks to automation and efficient false alarm filtering technologies.
There is always a chance of fires, explosions, and spills in the oil and gas business, putting the lives of workers at risk due to the intense working conditions and dangerous practises that are standard in the industry. This means they have a lot of rules to follow to keep their employees safe at work. Algorithms developed for computer vision can help with on-the-fly site checks and tracking. In addition, the camera feed’s video analytics can confirm whether or not employees follow safety measures, such as donning protective gear and staying away from potentially hazardous areas.
Conclusion
When implemented, computer vision solutions can offer several benefits from improving worker safety, lowering operational expenses to optimizing core processes in the Oil & Gas sector. Most oil and gas firms would need computer vision systems due to their critical nature and specific operational workflows. Improved maintenance, safety, management, life-cycle sustainability, quality, and operational efficiency are the primary goals of the latest computer vision applications in the oil and gas industry.